Paper: https://paper.wikicrow.ai
Code: https://github.com/Future-House/paper-qa
Today, we are announcing PaperQA2, the first AI agent to achieve superhuman performance on a variety of different scientific literature search tasks. PaperQA2 is an agent optimized for retrieving and summarizing information over the scientific literature. PaperQA2 has access to a variety of tools that allow it to find papers, extract useful information from those papers, explore the citation graph, and formulate answers. PaperQA2 achieves higher accuracy than PhD and postdoc-level biology researchers at retrieving information from the scientific literature, as measured using LitQA2, a piece of the LAB-Bench evals set that we released earlier this summer. In addition, when applied to produce wikipedia-style summaries of scientific information, WikiCrow, an agent built on top of PaperQA2, produces summaries that are more accurate on average than actual articles on Wikipedia that have been written and curated by humans, as judged by blinded PhD and postdoc-level biology researchers.
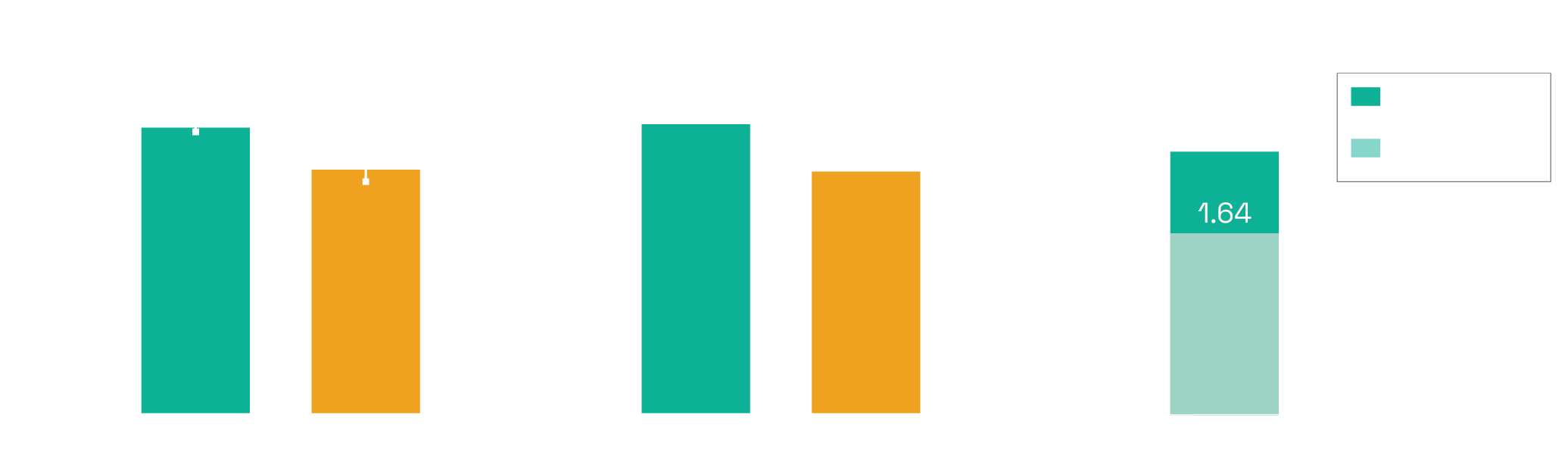
PaperQA2 allows us to perform analyses over the literature at a scale that are currently unavailable to human beings. Previously, we showed that we could use an older version (PaperQA) to generate a Wikipedia article for all 20,000 genes in the human genome, by combining information from 1 million distinct scientific papers. However, those articles were less accurate on average than existing articles on Wikipedia. Now that the articles we can generate are significantly more accurate than Wikipedia articles, one can imagine generating Wikipedia-style summaries on demand, or even regenerating Wikipeda from scratch with more comprehensive and recent information. In the coming weeks, we will use WikiCrow to generate Wikipedia articles for all 20,000 genes in the human genome, and will release them at wikicrow.ai. In the meantime, wikicrow.ai contains a preview of 240 articles used in the paper.
In addition, we are very interested in how PaperQA2 could allow us to generate new hypotheses. One approach to that problem is to identify contradictions between published scientific papers, which can point the way to new discoveries. In our paper, we describe how ContraCrow, an agent built on top of PaperQA2, can evaluate every claim in a scientific paper to identify any other papers in the literature that disagree with it. We can grade these contradictions on a Likert scale to remove trivial contradictions. We find 2.34 statements per paper on average in a random subset of biology papers that are contradicted by other papers from anywhere else in the literature. Exploring these contradictions in detail may allow agents like PaperQA2 and ContraCrow to generate new hypotheses and propose new pivotal experiments.

This is the very beginning of a new way of interacting with and extracting information from the scientific literature. There will be much more to come on this soon.